Introduction
In personalized medicine, multi-omics present potential that can be explored through ML methods, including “black-box” models, such as deep neural networks, to generate predictions and knowledge about domain relationships contained in data.
The inability to explain their results to clinical experts in a human-understandable way hinders their usability in the medical domain.
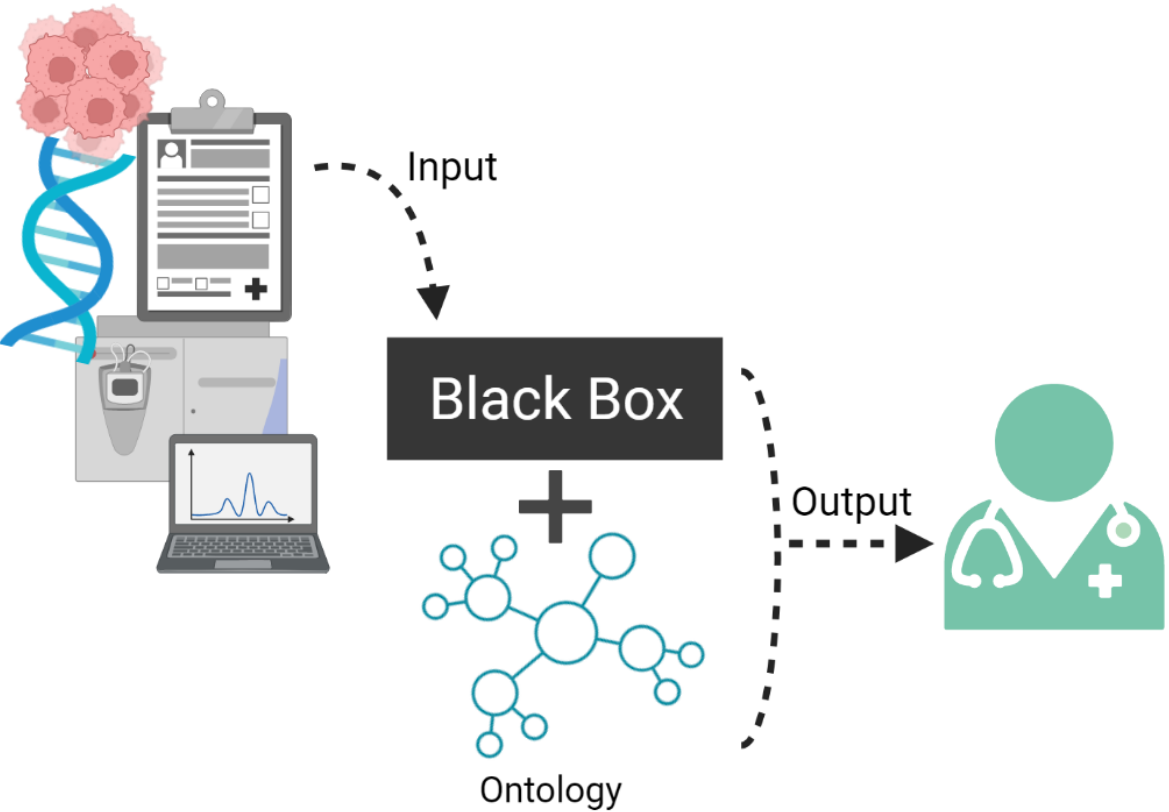
There is a possible solution for this problem in adding explainability to learning algorithms, by providing a contextual semantic layer through ontologies and Knowledge Graphs (KG).
This will allow to bridge the gap between AI data and medical application, by making medical “AI-empowered knowledge” accessible for clinicians and clinical researchers to understand and use.