Motivation
Artificial intelligence (AI) and Machine Learning (ML) have been achieving great results in the biomedical domain. There are valuable deep learning approaches with promising results, but they are “black-box” - their models are uninterpretable by humans. Their lack of explainability severely limits their trustability, specially in sensitive fields, where errors in predictions can have very damaging outcomes. So, there is a growing interest in the field of explainable AI (XAI).
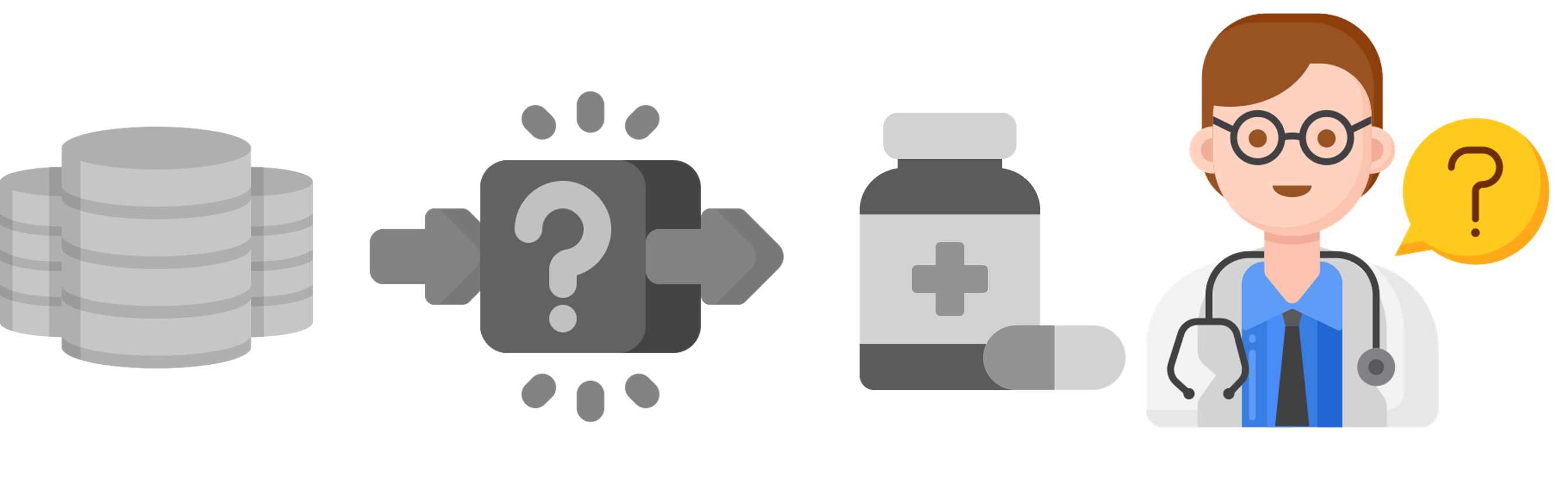
Semantic Explanations emerge as a strategy for explainable AI applications. They use Ontologies and Knowledge Graphs (KG), which model data and represent it in a connected structure. KG and their semantic context can be used for explainable AI in several different ways, such as to encode context, to better organize features, or to provide a semantic layer to explanations.
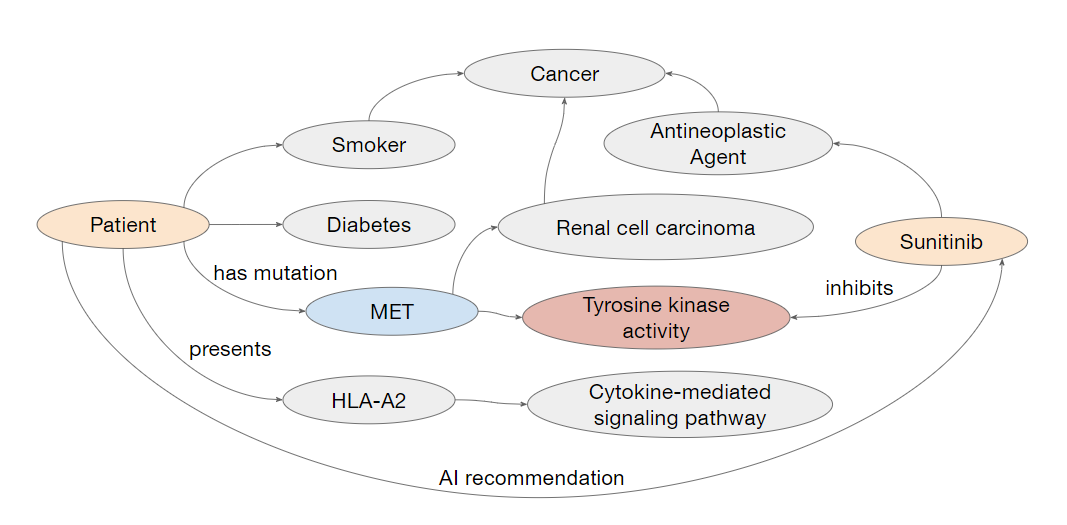
Our goal is to investigate how visualizations can support XAI for drug therapy recommendations in personalized oncology in the context of the KATY project.
Filter relevant paths in the KG that connect a patient to their predicted drug Design a visualization approach to increase understanding of explanations for AI predictions
Design a visualization approach to increase understanding of explanations for AI predictions